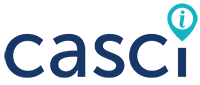
Dr. Luther gave an invited presentation at the Center for the Advanced Study of Communities and Information (CASCI) at the University of Maryland on November 19, 2019. The title of his presentation was, “Crowd Sleuths: Solving Mysteries with Crowdsourcing, Experts and AI.” The abstract was as follows:
Professional investigators in fields such as journalism, law enforcement, and academia have long sought the public’s help in solving mysteries, typically by providing tips. However, as social technologies capture more digital traces of daily life and enable new forms of collaboration, members of the public are increasingly leading their own investigations. These efforts are perhaps best known for high-profile failures characterized by sloppy research and vigilantism, such as the 2013 Boston Marathon Bombing manhunt on Reddit and 4chan. However, other crowdsourced investigations have led to the successful recovery of missing persons and apprehension of violent criminals, suggesting real potential. I will present three projects from my research group, the Crowd Intelligence Lab, where we helped to enable novice crowds to discover a hidden terrorist plot within large quantities of textual evidence documents; collaborate with expert investigators to geolocate and verify (or debunk) photos and videos shared on social media; and use AI-based face recognition to identify unknown soldiers in historical portraits from the American Civil War era.