Photo Sleuth: Identifying Historical Portraits with Crowdsourcing and Computer Vision
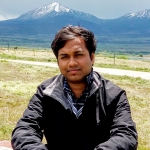
This NSF-funded project, a collaboration between Computer Science, History, and Military Images magazine, seeks to recover the lost identities of portraits of the American Civil War generation. The popularity of photography exploded in the United States during the 1860s as Americans went off to war. Today, 150 years later, thousands of these photos have survived, but relatively few are identified to particular individuals. We are developing new techniques combining crowdsourcing and computer vision, including face recognition technology, to piece together visual clues from photographs and research from historical sources to solve these mysteries. Visit CivilWarPhotoSleuth.com for details.
Selected Papers
Second Opinion: Supporting last-mile person identification with crowdsourcing and face recognition
Vikram Mohanty, Kareem Abdol-Hamid, Courtney Ebersohl, Kurt Luther
HCOMP 2019 Paper
Photo Sleuth: Combining human expertise and face recognition to identify historical portraits
Vikram Mohanty, David Thames, Sneha Mehta, Kurt Luther
IUI 2019 Paper Best Paper Award
Are 1,000 Features Worth A Picture? Combining Crowdsourcing and Face Recognition to Identify Civil War Soldiers
Vikram Mohanty, David Thames, Kurt Luther
HCOMP 2018 Demo Best Poster/Demo Award
GroundTruth: Supporting Image Geolocation with Crowdsourcing and Diagramming
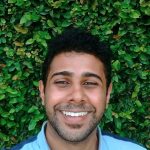
This NSF-funded project investigates how crowdsourcing can be used to identify or verify the specific geographic location where photos were taken. For example, a journalist may need to verify photos posted on social media for a new story, an intelligence analyst may need to track down the location of a terrorist training camp, or a museum archivist may need to identify historical photos in their collections. We are studying how novices and experts perform image geolocation tasks in order to understand the mental models they create, the image clues they use, and the challenges they face. We are also developing new software tools that leverage crowdsourcing and expert diagrams to support faster, more accurate image geolocation in a variety of domains.
Selected Papers
GroundTruth: Augmenting expert image geolocation with crowdsourcing and shared representations
Sukrit Venkatagiri, Jacob Thebault-Spieker, Rachel Kohler, John Purviance, Rifat Sabbir Mansur, Kurt Luther
CSCW 2019 Paper
Supporting Image Geolocation with Diagramming and Crowdsourcing
Rachel Kohler, John Purviance, Kurt Luther
HCOMP 2017 Paper Notable Paper Award
An Exploratory Study of Human Performance in Image Geolocation Tasks
Sneha Mehta, Chris North, Kurt Luther
HCOMP 2016 Workshop Paper
PairWise: Supporting Trustworthy Gig Work with Crowdsourced Observation
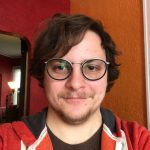
This NSF-funded project investigates how crowdsourced observation can be used to support trustworthy work in online work settings. For example, content moderation systems need crowd workers to make unbiased moderation decisions, or citizen science systems need to ensure a that citizen scientists follow a trustworthy scientific protocol. We are studying how having a real-time observer affects and changes online work behavior, the feasibility of this approach, and the potential to support less biased and more trusthworthy online work. We are also developing new software tools that enable this kind of interaction, as well as optimize the process (e.g., through opportunistically focusing a real-time observer on certain types of work).
Selected Paper
PairWise: Mitigating political bias in crowdsourced content moderation
Jacob Thebault-Spieker, Sukrit Venkatagiri, David Mitchell, Chris Hurt, Kurt Luther
HCOMP 2019 Demo Best Poster/Demo Award
CrowdIA: Supporting Intelligence Analysis with Crowdsourcing and Context Slices
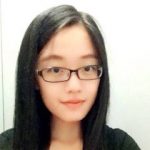
This NSF-funded project explores how crowdsourcing can be used to help an intelligence analyst find connections within a large body of text-based evidence. For example, an analyst may have access to dozens of evidence documents, and needs to identify a hidden terrorist plot that links the evidence together. We have developed the concept of “context slices,” in which we intelligently divide up large amounts of text so that transient, novice crowd workers can contribute to solving the bigger mystery. From these ideas, we have developed CrowdIA, a web-based system that supports solving mysteries in text documents using crowdsourced sensemaking.
Selected Papers
CrowdTrace: Visualizing provenance in distributed sensemaking
Tianyi Li, Yasmine Belghith, Chris North, Kurt Luther
VIS 2020 Paper
Dropping the baton? Understanding errors and bottlenecks in a crowdsourced sensemaking pipeline
Tianyi Li, Chandler J. Manns, Chris North, Kurt Luther
CSCW 2019 Paper
CrowdIA: Solving mysteries with crowdsourced sensemaking
Tianyi Li, Kurt Luther, Chris North
CSCW 2018 Paper
CrowdLayout: Improving Biological Graph Visualizations with Crowdsourcing
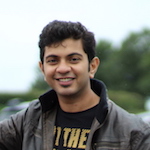
This NIH-funded project investigates how crowdsourced design can be used to improve visualizations of biological graph data. Many types of life sciences research generate graph data, but meaningful visualizations are hard to achieve with automatic graph layout algorithms, and time-consuming for experts to create manually. We have developed CrowdLayout (formerly GraphCrowd), a system that allows novice crowd workers to design and review biological network layouts as effectively as experts. CrowdLayout is an extension of GraphSpace, an online hub developed at Virginia Tech where researchers can easily share and visualize biological network data.
Selected Papers
Flud: A hybrid crowd-algorithm approach for visualizing biological networks
Aditya Bharadwaj, David Gwizdala, Yoonjin Kim, Kurt Luther, T.M. Murali
CHI 2019 Workshop Paper
CrowdLayout: Crowdsourced Design and Evaluation of Biological Network Visualizations
Divit P. Singh, Lee Lisle, T. M. Murali, Kurt Luther
CHI 2018 Paper
GraphSpace: Stimulating Interdisciplinary Collaborations in Network Biology.
Aditya Bharadwaj, Divit P. Singh, Anna Ritz, Allison N. Tegge, Christopher L. Poirel, Pavel Kraikivski, Neil Adames, Kurt Luther, Shiv D. Kale, Jean Peccoud, John J. Tyson, T. M. Murali
Bioinformatics (2017)
Incite: Supporting Historical Research and Education with Crowdsourcing
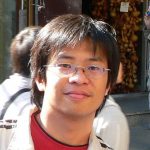
This interdisciplinary collaboration between Computer Science, History, and Education investigates how a crowdsourcing system can support historical scholarship while helping crowd workers learn about history. We created Incite, a system that combines crowdsourcing with natural language processing techniques to help expert scholars find relevant primary sources in an digital archive. Incite was developed as a plug-in for the open-source Omeka content management system used by many museums and galleries. We have deployed Incite in high school and college classrooms across the US as part of two digital humanities projects, Mapping the Fourth of July in the Civil War Era (funded by the National Archives), and The American Soldier in World War II (funded by the NEH).
Selected Papers
Read-Agree-Predict: A crowdsourced approach to discovering relevant primary sources for historians
Nai-Ching Wang, David Hicks, Paul Quigley, Kurt Luther
Human Computation Journal (2019)
Exploring Trade-Offs Between Learning and Productivity in Crowdsourced History
Nai-Ching Wang, David Hicks, Kurt Luther
CSCW 2018 Paper
Crowdnection: Connecting High-level Concepts with Historical Documents via Crowdsourcing
Nai-Ching Wang
CHI 2016 Student Research Competition