A commitment to innovation and sustainability
Études is a pioneering firm that seamlessly merges creativity and functionality to redefine architectural excellence.
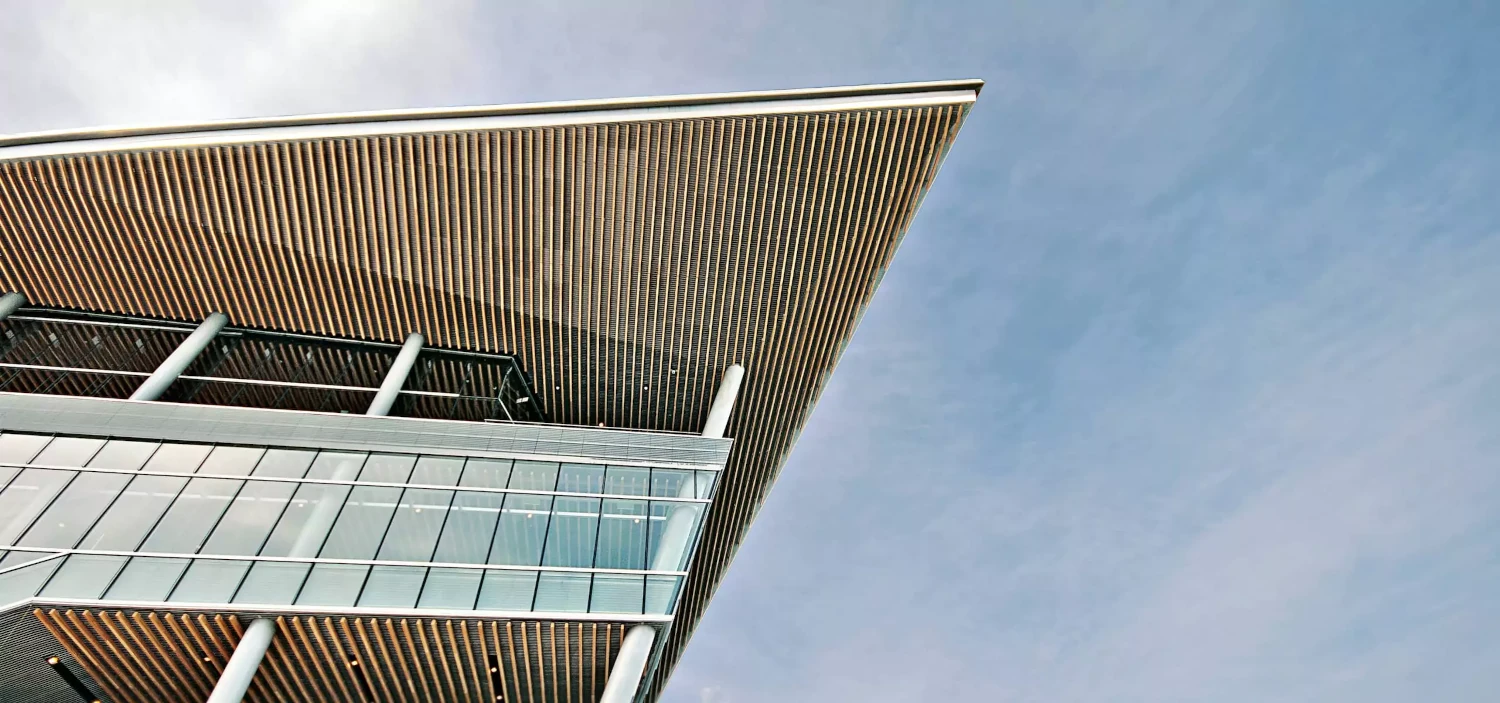
A passion for creating spaces
Our comprehensive suite of professional services caters to a diverse clientele, ranging from homeowners to commercial developers.
Renovation and restoration
Experience the fusion of imagination and expertise with Études Architectural Solutions.
Continuous Support
Experience the fusion of imagination and expertise with Études Architectural Solutions.
App Access
Experience the fusion of imagination and expertise with Études Architectural Solutions.
Consulting
Experience the fusion of imagination and expertise with Études Architectural Solutions.
Project Management
Experience the fusion of imagination and expertise with Études Architectural Solutions.
Architectural Solutions
Experience the fusion of imagination and expertise with Études Architectural Solutions.
An array of resources
Our comprehensive suite of professional services caters to a diverse clientele, ranging from homeowners to commercial developers.
Études Architect App
- Collaborate with fellow architects.
- Showcase your projects.
- Experience the world of architecture.
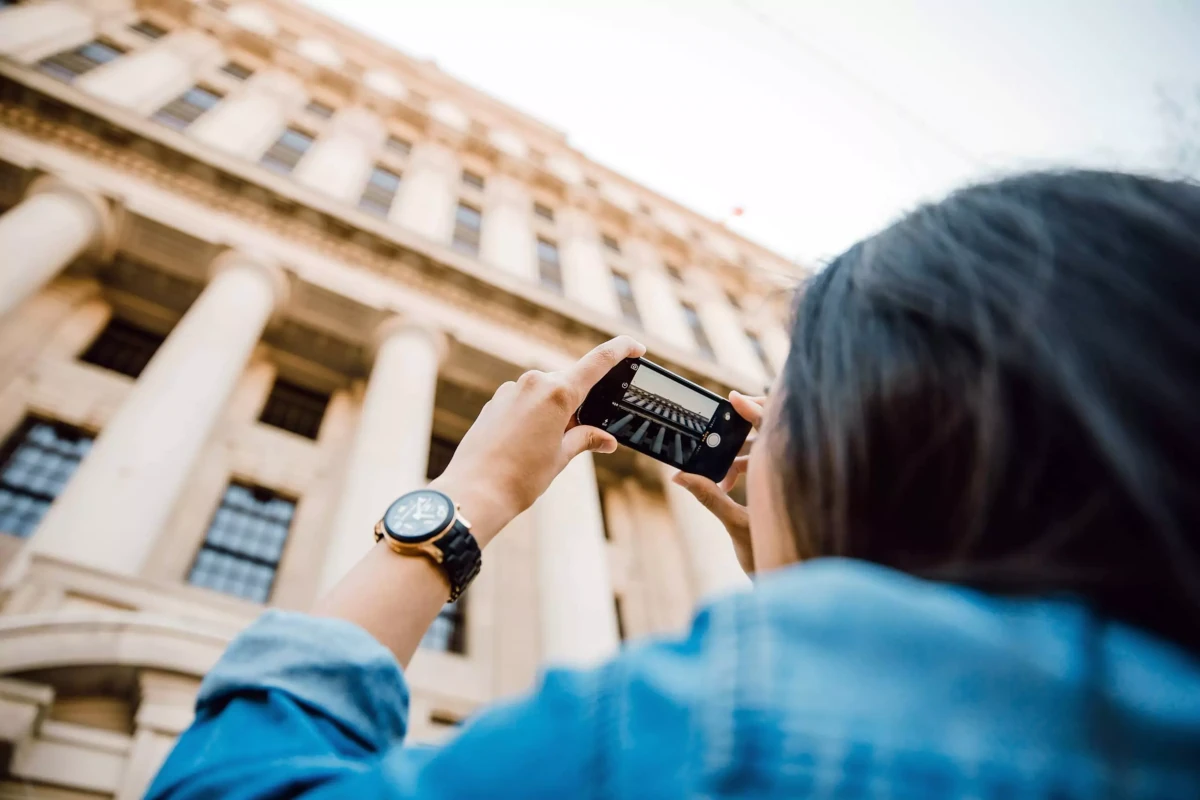
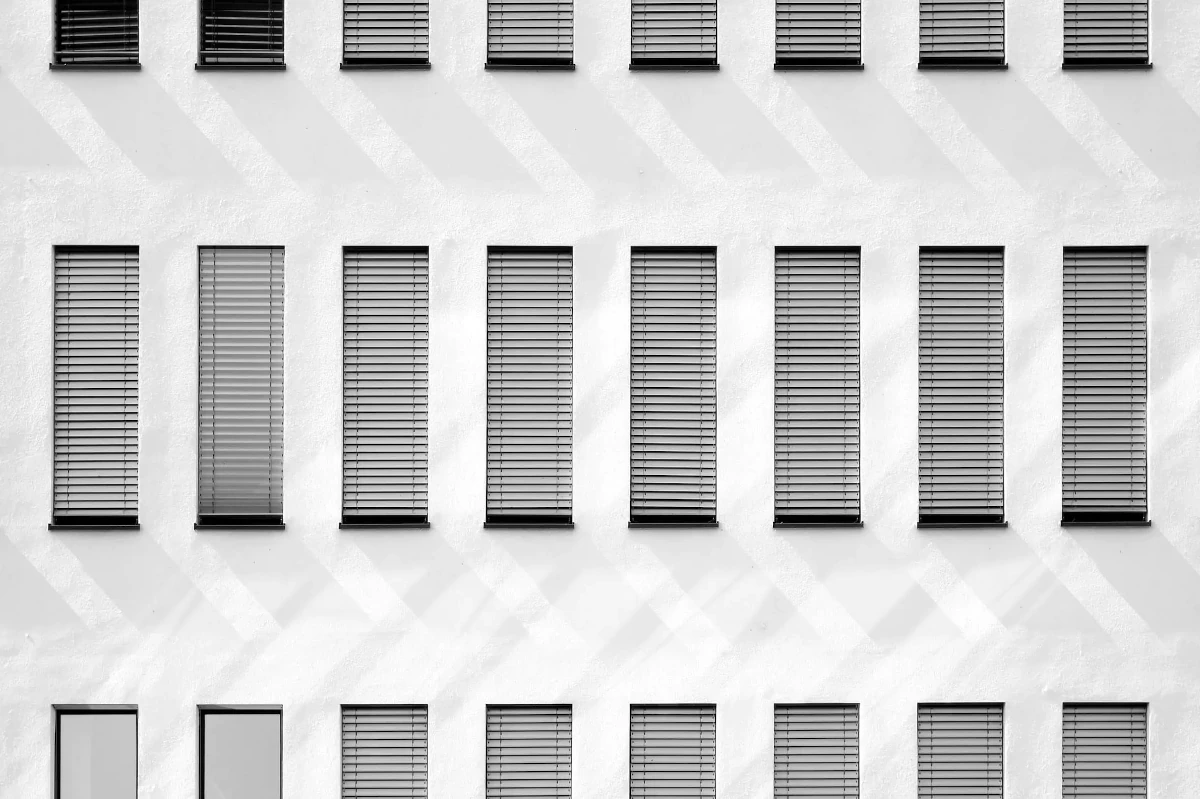
Études Newsletter
- A world of thought-provoking articles.
- Case studies that celebrate architecture.
- Exclusive access to design insights.
“Études has saved us thousands of hours of work and has unlocked insights we never thought possible.”
Annie Steiner
CEO, Greenprint
Watch, Read, Listen
Join 900+ subscribers
Stay in the loop with everything you need to know.