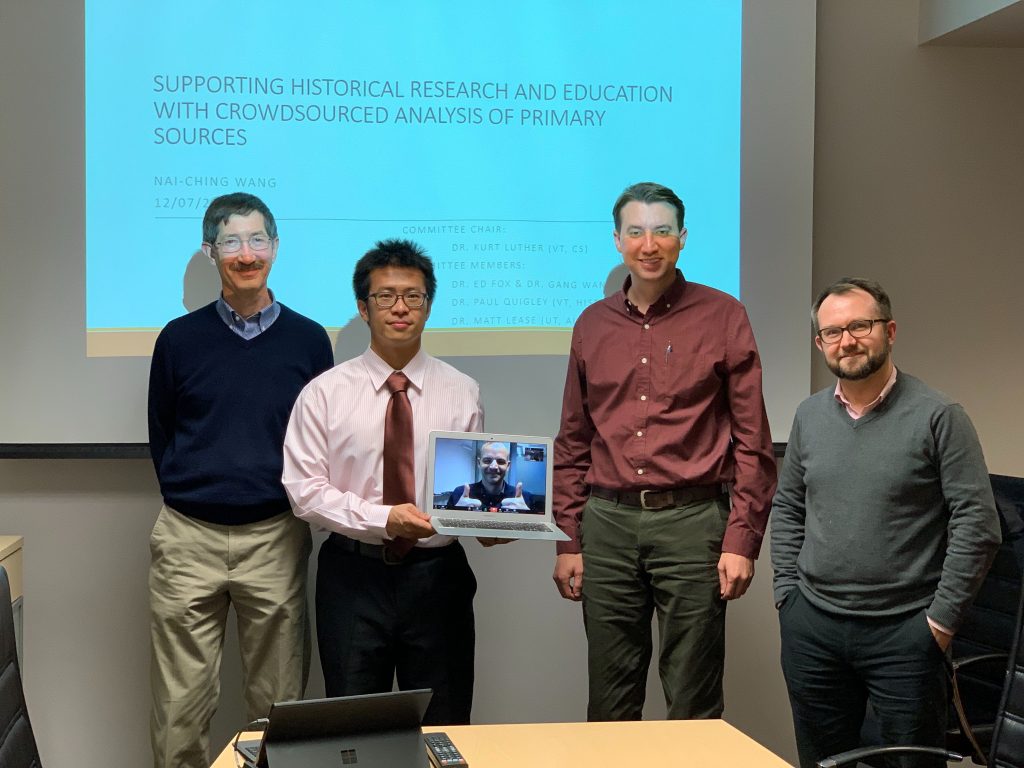
Nai-Ching Wang, a Ph.D. student advised by Dr. Luther, successfully defended his dissertation today. His dissertation is titled, “Supporting Historical Research and Education with Crowdsourced Analysis of Primary Sources”, and his committee members were Dr. Luther (chair), Ed Fox, Gang Wang, and Paul Quigley, with Matt Lease (UT Austin School of Information) as the external member. Here is the abstract for his dissertation:
Historians, like many types of scholars, are often researchers and educators, and both roles involve significant interaction with primary sources. Primary sources are not only direct evidence for historical arguments but also important materials for teaching historical thinking skills to students in classrooms, and engaging the broader public. However, finding high quality primary sources that are relevant to a historian’s specialized topics of interest remains a significant challenge. Automated approaches to text analysis struggle to provide relevant results for these “long tail” searches with long semantic distances from the source material. Consequently, historians are often frustrated at spending so much time on manually the relevance of the contents of these archives other than writing and analysis. To overcome these challenges, my dissertation explores the use of crowdsourcing to support historians in analysis of primary sources. In four studies, I first proposed a class-sourcing model where historians outsource historical analysis to students as a teaching method and students learn historical thinking and gain authentic research experience while doing these analysis tasks. Incite, a realization of this model, deployed in 15 classrooms with positive feedback. Second, I expanded the class-sourcing model to a broader audience, novice (paid) crowds and developed the Read-agree-predict (RAP) technique to accurately evaluate relevance between primary sources and research topics. Third, I presented a set of design principles for crowdsourcing complex historical documents via the American Soldier project on Zooniverse. Finally, I developed CrowdSCIM to help crowds learn historical thinking and evaluated the tradeoffs between quality, learning and efficiency. The outcomes of the studies provide systems, techniques and design guidelines to 1) support historians in their research and teaching practices, 2) help crowd workers learn historical thinking and 3) suggest implications for the design of future crowdsourcing systems.
Congratulations Dr. Wang!